Large language models outshine traditional natural language processing methods for identifying rare circumstances
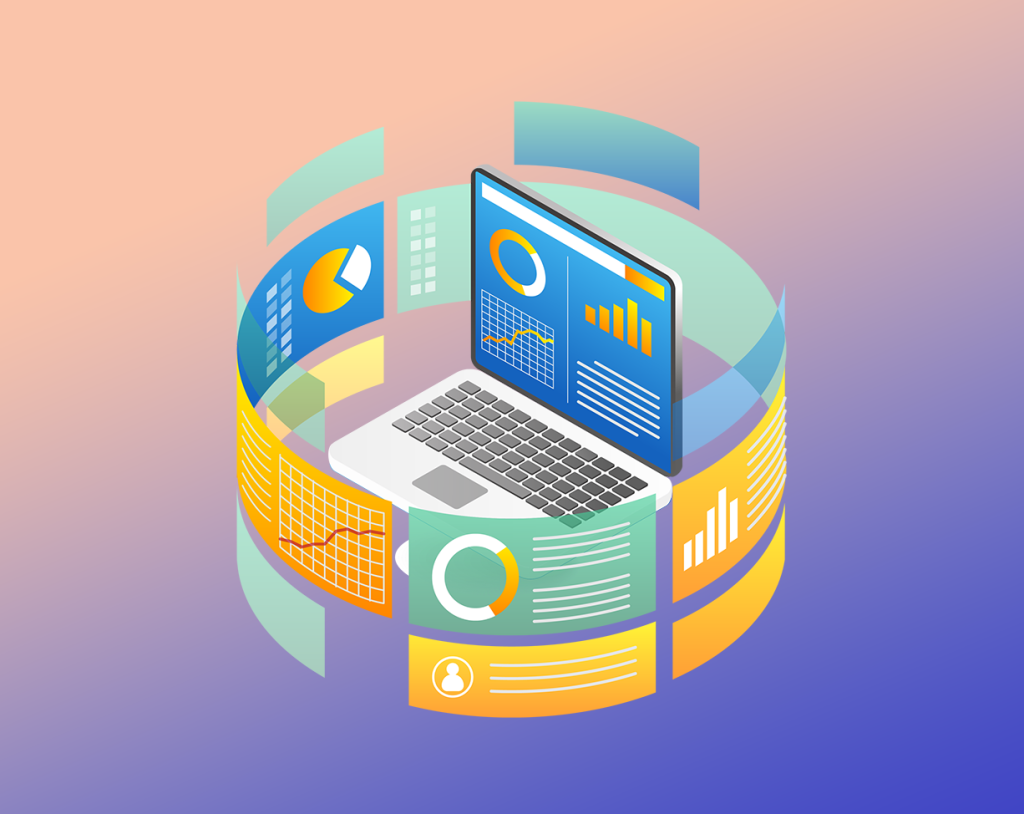
Researchers have recently begun using Natural Language Processing (NLP) to analyze case files and other large batches of information more efficiently. However, traditional NLP requires a human to review case files and train the program to recognize nuanced or ambiguous language. For example, when a report says, “The patient reported that their head hurt,” the researcher wants NLP to recognize this as a headache, even though the report doesn’t use the word “headache.” Training the NLP to recognize such nuance requires manually reviewing enough case files with nuanced language to allow the NLP to learn the language patterns. This review is labor intensive and may not identify enough cases when circumstances are rare. Researchers wanted to know: Could a large language model—the artificial intelligence (AI) technology also used by ChatGPT—be trained to identify rare circumstances in large amounts of unstructured data?
The National Violent Death Reporting System (NVDRS) provides a wealth of information on violent deaths, offering unstructured incident narrative reports from coroners, medical examiners, and law enforcement. In a recent study, researchers from the University of Washington Department of Epidemiology used this database to test large language models by utilizing reports on female firearm suicides and the nuanced circumstances preceding them.
Using 1,462 reports from the NVDRS, researchers leveraged a large language model to analyze the narratives from each report. Differing from the traditional NLP approaches, the large language model does not require any annotated reports for training. The researchers focused on circumstances preceding female firearm suicides that did not occur often in NVDRS reports (e.g., sexual violence was noted as a precipitating factor in 38 of the 1462 reports) and asked the language models yes or no questions to test whether the model identified these circumstances. The large language models performed surprisingly well. One model called FLAN-UL2, originally developed by Google, can follow complex instructions as well as perform competitively in comprehension and arithmetic and causal reasoning.
“With the traditional NLP approach, researchers need to manually annotate a subset of reports. Only then can they train the machine learning model to do the automatic annotations,” says UW Epidemiology PhD student Weipeng Zhou, who was the lead researcher on the study. “But large language models are gradually relaxing this constraint.” The results of this study show promise in avoiding the intensive human labor to text mine for the language that NLP can’t catch. AI language models like FLAN-UL2 can not only be trained, but can outperform NLP by a wide margin, in some scenarios where the traditional NLP struggles.
While male firearm suicide rates have historically been higher, female firearm suicide rates saw a startling increase of 20 percent between 2010-2020, surpassing the rate increase among males. The study focused on “infrequent circumstances” when testing AI language models. Infrequent circumstances are various issues that an individual may have been dealing with prior to their death, such as sleep problems, loneliness, custody issues, or bullying. In theory, tracking these issues could give researchers and healthcare professionals the key to understand the statistical spike in female firearm suicides. Dr. Stephen Mooney, Department of Epidemiology Assistant Professor, says that it is use of the tool for future public health implications that makes this study particularly exciting. “The challenge of doing a lot of work using plain text records of individual stories or clinical information is that it’s really hard to identify things that are very important but happen rarely. What we tested is just one scenario where there are rare antecedent events that you can mine from text, but there are lots of scenarios where there may be important factors discussed in a clinical narrative where we would have a hard time finding with conventional NLP to date,” Mooney describes. “The large language model already has context for what certain words mean and we can much more efficiently identify those rarer scenarios.” By using a chatbot and yes or no questions, researchers can better understand the data in thousands of reports, making the process altogether faster as well as more intuitive.
“People think of AI chatbots as something that can create language for humans, like writing an essay,” Mooney says. “I want people to be excited about their potential for use in deductive circumstances, too. It’s not just that these models can create new language, but also that they can classify information, or unlock data that is otherwise locked up.”
The study highlights the incredible potential of large language models in bridging the gap in gathering and analyzing unstructured information from many of these reports, not only in cases of female firearm suicides, but also unlocking critical insights for public health research. There are other ways large language models can be applied, such as a follow-up project being explored by Dr. Mooney and colleagues that tracks helmet use in bike and scooter collisions using reports from the emergency department. Language models can begin to help researchers analyze questions about helmet use or environmental factors that influence rider collisions. This approach offers an improvement over traditional methods, providing a valuable tool for researchers who, up until now, could only use NLP. While further exploration into the applications of AI language models is needed, their use could provide opportunities for future research or patient care.